AI and Revenue Management
by francesco d'acunto

When you are dealing with Revenue Management, but more generally when you have large amounts of data to manage, giving in to the lure of technology is really easy and, in some ways, necessary.
Just think of the speed of computation or the finding of associations in big data and it will be obvious how no human brain can ever beat a computer in speed and efficiency.
For example, analyzing a Hotel Cluster in a given destination involves processing and managing a large amount of data: think average price, booking window, occupancy, cancellation rate, room type, and more.
Putting this data together, but more importantly, interpreting it profitably, requires method and appropriate tools.
The method cannot do without statistics, which plays a key role in data analysis. Without its ability to synthesize, one of the most valued properties, it would be really complicated to notice that, in a particular period, some parameter of our Hotel is taking on anomalous characteristics. Here then, thanks to statistics, we can easily summarize all the data being analyzed into a few (for us) most significant values.
Tools for data analysis today there are really numerous and now more and more PMS are beginning to integrate Business Intelligence modules and Dashboards capable of grouping together some basic KPIs (Key Performance Indicators).
Having assumed that in order to improve not only the quality of his or her daily existence (but also his or her work performance) every Revenue Manager will use statistical basics and a few tools to support his or her work, let's see what happens when we perhaps start to get the hang of the concept of Artificial Intelligence.
For example, there have existed for many years PMSs that can change the sales rate range of our Hotel upon the occurrence of certain conditions that, normally, correspond to higher or lower occupancy or, again, to the simultaneous purchase of several rooms (which then always a change in occupancy is).
We tested them already years ago and quickly understood the limits of an automatism that operates following a rigid scheme.
Let's assume, for example, that our PMS (or an external module) has been programmed to set the price range higher upon the occurrence of an occupancy of more than 80 percent in our hotel.
In reality, the software does nothing more than count our occupancy and match it with a sales price (alas, even today this is one of the variants used to summarize a revenue manager's job).
But suppose we are in an off-season and, by pure chance, we were lucky enough to have a group reservation that occupied 80 percent of our hotel. Our competitors have a lot of availability-and consequently low rates-but for ours our software is almost full and so it publishes (as we asked it to do) a high rate thus minimizing the chances of sales.
So here is where someone will pronounce the solution to the problem: the algorithm.
In fact, by merging the data from my Hotel and the market data, here is where the AI software manages to calculate the exact formula that, in our case, will result in the best selling price.
Software based on algorithms and machine learning (because they suggest a rate to you, the human makes the final decision and the algorithm learns) are now popping up like mushrooms after a rainstorm.
Unfortunately, riding the wave a little bit of fashion, a little bit of misused words (I've heard people talking about logarithms suggesting rates), sometimes we also happen to witness some paradoxes such as salespeople approaching Hotel owners offering a few thousand euro software that, according to them, can replace the work of their Revenue Manager and grow revenue exponentially.
If a Hotel's Revenue is managed superficially (or unmanaged) it will certainly be likely to increase turnover with software that makes a pricing policy dynamic but let us reflect for a moment on the concrete application of AI to Revenue Management.
The contradiction starts from the very idea (which in some cases one has an interest in propagating) that Revenue can be summarized in the activity of Pricing (vulgarly making rates).
I say this not only to myself, but to many colleagues I respect: the "rates" stage is only the last, the final one in a long decision-making process supported by data analysis.
So, at the limit, this software would only go to support the last of all the logical activities previously performed by a human brain.
Why couldn't a tireless software that is active h24, that has historical and demand data available, that has learning capabilities replace a live Revenue Manager (who costs more and is lazier)?
On the other hand, if planes have been flying safely for a long time already thanks to algorithms, why shouldn't they be able to post a fare correctly?
Simple the question, equally simple the answer: the environment where an airliner operates is less complex than the sales environment of a Hotel.
Who better than we have learned in recent years that we operate in a business environment that is anything but stable and predictable?
Did anyone predict the anomalous August performance of some destinations compared with other summer months?
How does the algorithm interpret a slow pickup? Can it reason about a factor affecting the flow of reservations such as, for example, a different cancellation policy?
In the decision-making process of buying a room, how does it relate similar but different types of a competitor?
Here is the first serious problem: computing power is not capable of producing causal thinking.
Sure, the algorithm can compute numerous scenario possibilities much faster than our minds, but common sense poses a huge challenge for artificial intelligence.
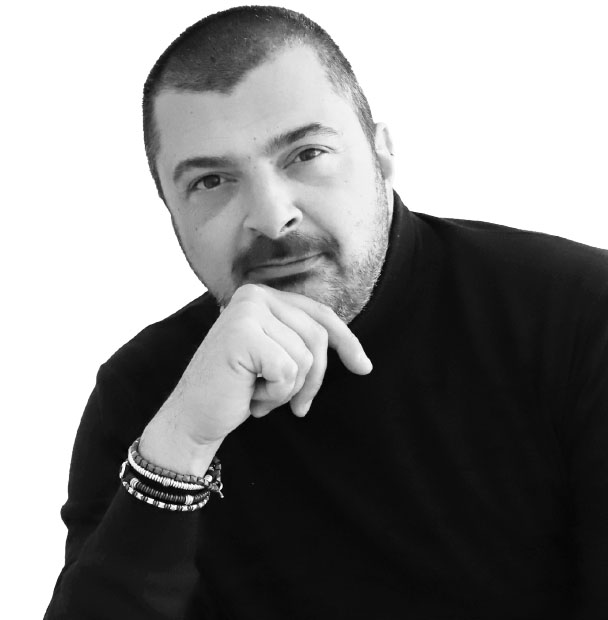